In the modern era, data is often referred to as the new oil. The ability to predict future trends and behaviours has become a key asset for businesses and organisations. Predictive analytics combines mathematics, big data, and machine learning. It is at the forefront of this transformation.
This guide looks at predictive analytics. It covers why it matters, its main parts, and how it changes decision-making in different industries. No matter if you’re a business leader, data analyst, or tech fan, knowing predictive analytics can help you make smart choices. These choices lead to success.
Why Predictive Analytics Matters
Predictive analytics is more than just a buzzword. It is a game-changer in data-driven decision-making. It uses statistical algorithms and machine learning to analyse past data and predict future results. In today’s fast-paced world, organisations must anticipate changes and adapt quickly.
Some key benefits of predictive analytics include:
- Boosting business performance: Spot inefficiencies and improvement areas. This leads to smoother operations and higher profits.
- Enhancing customer experience: This helps businesses grasp customer behavior and preferences. Then, they can tailor their offerings.
- Mitigating risks: This helps organisations spot and handle risks in finance, cybersecurity, and healthcare.
Companies can use predictive analytics to stay ahead and make better decisions.
Quick Guide: Using Predictive Analytics for Smarter Decision Making
- Predictive analytics helps businesses anticipate future trends by using data, statistical algorithms, and machine learning.
- By understanding past patterns, organisations can make informed, proactive decisions that enhance performance, customer satisfaction, and risk management.
Step-by-Step Guide to Implementing Predictive Analytics
Step 1: Define Clear Objectives
Before embarking on a predictive analytics project, clearly define what you aim to achieve. Examples of goals include:
- Increasing customer retention rates
- Optimising supply chain efficiency
- Reducing fraud and security risks
- Enhancing patient care in healthcare settings
Clearly outlining objectives ensures that predictive analytics efforts align with business priorities.
Step 2: Gather and Prepare Data
The accuracy of predictive models depends on the quality of the data used. Follow these best practices:
- Gather important data: Look at sales records, customer interactions, IoT sensor info, and market trends.
- Clean and preprocess data: Remove duplicates, fix missing values, and ensure data consistency.
- Store and manage data effectively: Utilise data warehouses or cloud-based storage solutions.
Step 3: Choose the Right Predictive Modeling Techniques
Several modelling techniques exist, each suited for different types of analysis:
- Regression Analysis: Used for forecasting trends such as sales, stock prices, and customer demand.
- Decision Trees: Helpful for classification problems, such as segmenting customers into groups.
- Neural Networks: These are advanced models in machine learning. They handle complex tasks, such as image recognition and fraud detection.
Selecting the right technique depends on the nature of the problem and the type of data available.
Step 4: Train and Validate Models
Once a model is selected, it must be trained on historical data and tested to ensure accuracy. Best practices include:
- Splitting data: Divide data into training and testing sets to evaluate performance.
- Cross-validation: Use multiple rounds of training and testing to avoid overfitting.
- Adjusting model parameters: Optimise algorithms to improve predictive accuracy.
Step 5: Deploy and Monitor Models
After validation, predictive models are deployed for real-world use. Continuous monitoring is essential to:
- Detect shifts in data patterns
- Update models with new data
- Ensure predictions remain accurate over time
Automated tools and dashboards help track performance and refine predictive analytics strategies.
Pro Tip: Start small by defining clear business goals for predictive analytics, such as improving customer retention or optimizing supply chains, and build from there
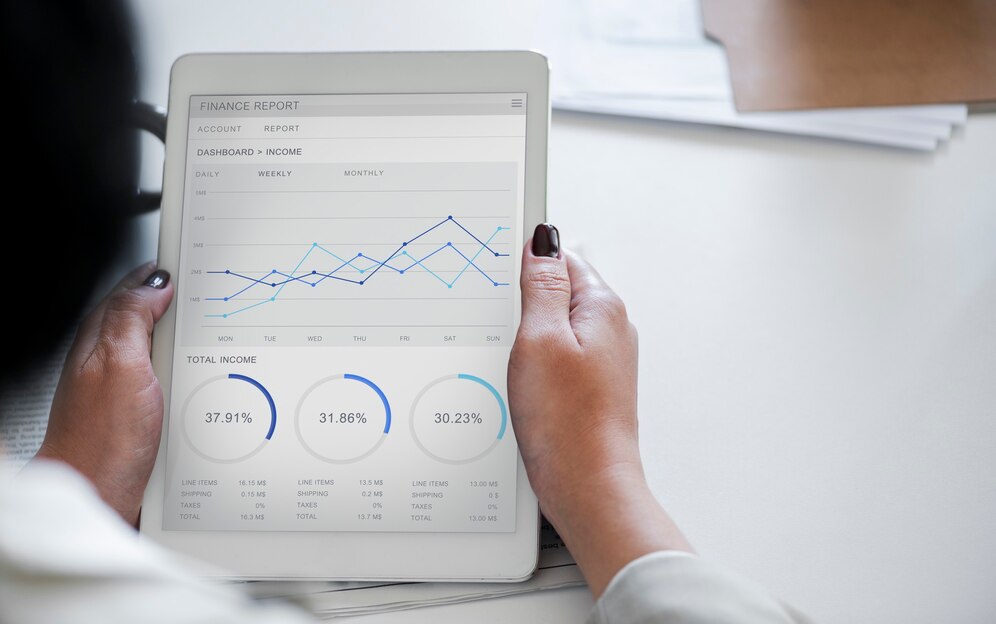
Common Challenges and How to Overcome Them
1. Overfitting Models
- Overfitting occurs when a model is too complex and captures noise instead of real patterns.
- Solution: Use techniques such as cross-validation and regularisation to improve model generalisation.
2. Lack of Quality Data
- Poor-quality data leads to inaccurate predictions.
- Solution: Invest in data cleaning, normalisation, and integration to ensure reliable inputs.
3. Ignoring Ethical Considerations
- Bias in predictive analytics can lead to unfair outcomes.
- Solution: Use diverse datasets and ensure transparency in model decision-making.
Advanced Insights: The Role of Machine Learning and AI
Machine learning is a key driver of predictive analytics advancements. It enables computers to analyse vast datasets, learn from patterns, and improve predictions over time.
Types of Machine Learning in Predictive Analytics
- Supervised Learning: Uses labeled data for training. It’s often used in fraud detection and medical diagnosis.
- Unsupervised Learning: It discovers hidden patterns in data. This helps with customer segmentation and market analysis.
- Reinforcement Learning: It uses rewards and penalties to improve decisions. You can find it in robotics and game theory.
Machine learning and predictive analytics work together. This combination helps industries make better and faster decisions.
Important: Quality data is essential for accurate predictions. Always ensure data is clean, relevant, and up-to-date before applying predictive models.
Frequently Asked Questions (FAQ)
What industries benefit the most from predictive analytics?
Predictive analytics is widely used in:
- Healthcare: Predicting patient outcomes and optimising treatment plans.
- Finance: Identifying fraudulent transactions and assessing credit risks.
- Retail: Forecasting demand and personalising marketing strategies.
- Manufacturing: Predicting equipment failures to reduce downtime.
- Cybersecurity: Detecting anomalies and preventing security breaches.
How accurate are predictive analytics models?
The accuracy depends on the quality of data, choice of algorithms, and continuous refinement. High-quality models can achieve accuracy rates of 80-95% in well-defined use cases.
Do I need coding skills to use predictive analytics?
Not necessarily. Tools like Tableau, Power BI, and cloud-based AI platforms are easy to use. They need little to no coding. However, knowledge of Python or R can be beneficial for advanced analytics.
What are some tools used in predictive analytics?
Popular tools include:
- Python & R: Used for statistical modelling and machine learning.
- IBM Watson & Google AI: Cloud-based AI solutions.
- Tableau & Power BI: Data visualisation and reporting.
- SAP Predictive Analytics: Enterprise-level analytics software.
How do businesses get started with predictive analytics?
Start by identifying key business problems that predictive analytics can solve. Invest in data tools and analytics. Hire skilled professionals. Also, refine models based on real-world results.
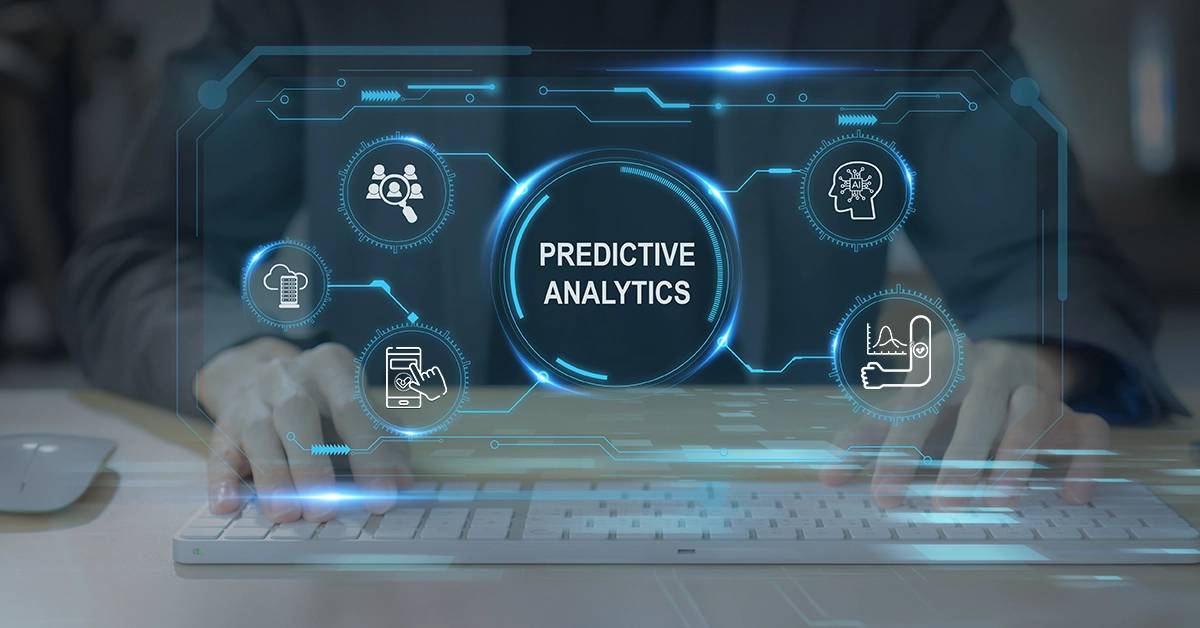
The Future of Predictive Analytics
Predictive analytics is changing how organisations decide. It helps them foresee future trends. Big data, machine learning, and advanced modelling help businesses find insights. These insights drive growth and spark innovation.
As technology advances, the potential for predictive analytics will only expand. Organisations that use these capabilities can better handle challenges, grab new chances, and stay competitive.
Are you ready to harness the power of predictive analytics for smarter decision-making? Start today and unlock the potential of data-driven insights!